Bio Statistics 분야에서 많이 활용되는 개념인 Propensity Score에 대해서 정리하고자 한다.
0. Propensity Score가 필요한 상황
Clinical research 분야에서 Randomized Controlled Trials(RCT)는 꽤 중요한 개념이다. 어떤 집단 A에 treatment에 대한 효과를 통계적으로 검증한다고 했을 때, 가장 이상적인 방법은 집단 A를 반으로 나눠 한쪽은 control group으로, 한쪽은 treatment를 진행한 group으로 나눠 수치를 얻어내는 것이다. 하지만 의료 분야에서는 현실적이지 않을 뿐더러 윤리적으로도 수행할 수 없는 경우가 많다. 흡연자에 대한 treatment를 검증하고자 할 때, 강제적으로 흡연을 시킬 수 없으니 말이다. 때문에 이미 실험적(experimental) 데이터가 아닌 관찰된(observational) 데이터를 주로 사용하게 된다. Propensity Score는 observational data를 가지고 causal inference를 할 때 집단간의 불균형으로 인한 bias를 보정하기 위해 사용이 된다. 그리고 보정된 값을 활용하여 Average Treatment Effect(ATE)를 계산한다.
*Experimental Data & Observational Data
Experimental data(e.g. AB test) is data collected as part of an AB test, such as a randomized experiment, controlled study or randomized control trials, among other variants. In an AB test, treatments are randomly assigned to the individuals and not selected by them. This means there is no selection bias and the treatment assignment is independent from any confounders.
Any data that is not collected as part of an AB test is observational data. In observational data, treatments are selected by the individuals and not randomly assigned to them. This means there is selection bias, and treatment is no longer independent from the confounding factors.
1. Propensity Score 개념
(1) 관련 용어 설명
Propensity Score를 설명하기 위해서 먼저 관련 용어를 정리하고자 한다.
- Outcome variable: A variable of interest for which we want to understand treatment’s effect on. This is the effect.
- Treatment variable: A variable of interest for which we want to understand its impact on the outcome variable. This is the cause.
- Confounding variable: A variable that influences both the selection of treatment and the outcome variables. In some contexts, this is also referred to as a covariate.

위와 같이 smoker, treatment, dead 항목으로 이루어진 데이터가 있다고 하자. 이때 단순히 treatment에 따른 사망 비율로 ATE를 아래와 같이 계산해보자.
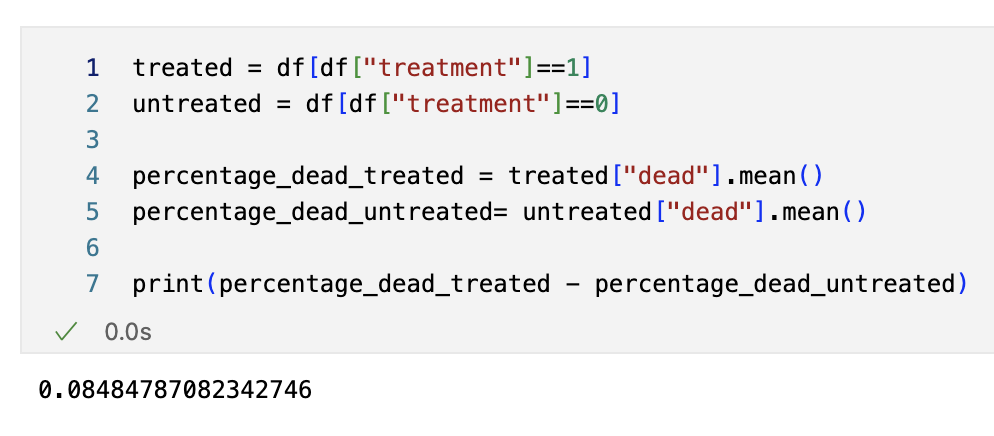
treated 집단의 사망률이 untreated 집단의 사망률보다 8.5% 높다는 결과가 나온다. 뭔가 이상하다. 이는 smoker라는 변인을 고려하지 않았기 떄문이다. Confounding variable(a.k.a covariate)은 이렇든 파악하고자 하는 cause외의 변인을 설명하기 위한 개념이다.
Basically, what this word means is that there is a variable or a group of them (the confounders) that affects in some way the probability of getting the treatment and at the same time, affects also the outcome.
하지만 confounder는 매우 많은 변인을 포함하고 있을 수 있는데, Propensity Score의 목적은 수 많은 변인들을 합쳐 하나의 score로 표현하는 것이다.
Propensity의 정의는 다음과 같다.
The "propensity" describes how likely a unit is to have been treated, given its covariate values. The stronger the confounding of treatment and covariates, and hence the stronger the bias in the analysis of the naive treatment effect, the better the covariates predict whether a unit is treated or not. By having units with similar propensity scores in both treatment and control, such confounding is reduced. (출처: 위키)
어떤 사람이 특정 confounder 변인 조합을 가지고 있을 때 treatment를 받았을 확률이다. 말이 조금 이상한데, 애초에 관찰된 데이터가 random하게 분포가 되지 않았으니, Propensity Score를 사용하여 비슷한 상황(confounder)인 사람들을 모아서 효과를 파악할 수 있다.
(2) Propensity Score 수식
ATE를 계산하기 위해서는 treated 집단과 untreated 집단의 효과 차이를 계산해야 한다.
$$P(Y=1|\text{do}(X=1)) - P(Y=1|\text{do}(X=0))$$
이때 $Z$가 주어졌을 때 $X,Y$의 관계를 설명할 수 있는 adjustment formula는 다음과 같다.
$$P(Y=y|\text{do}(X=x)) = \sum_{z}P(Y=y|X=x,Z=z)P(Z=z)$$
이때 Propensity Score는 다음과 같다(자세한 설명은 여기를 참고)
$$g(x,z) = P(X=x|Z=z)$$
위의 예시 데이터로는 다음과 같이 표현할 수 있다.
$$g(\text{smoker}) = P(\text{treatment}=1|\text{smoker})$$
Estimate of the function $g(x,z) = P(X=x|Z=z)$, often called the “propensity score,” for each $x$ and $z$, can be obtained by fitting the parameters of a flexible function $g(x,z)$ to the data at hand, in much the same way that we fitted the coefficients of a linear regression function, so as to minimize the mean square error with respect to a set of samples. The method used will depend on the nature of the random variable X, whether it is continuous, discrete or binary, for example.
위 수식을 토대로 예시 데이터의 Propensity Score를 계산하면 다음과 같다.
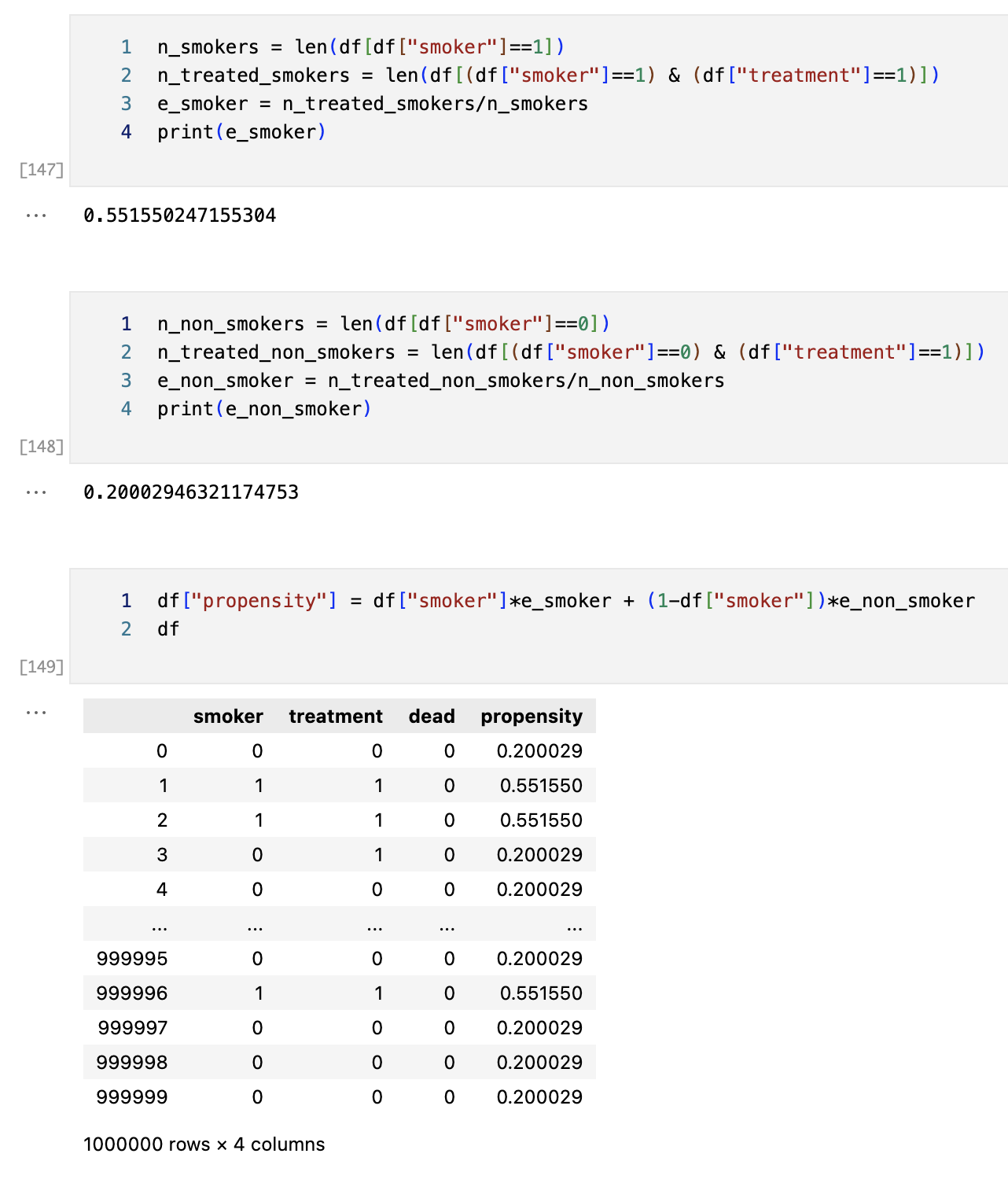
위 데이터로 ATE를 계산하면 다음과 같이 $-0.083$을 얻을 수 있으며, 이는 treated 집단이 untreated 집단보다 사망률이 약 8.3% 낮다는 것을 의미한다.
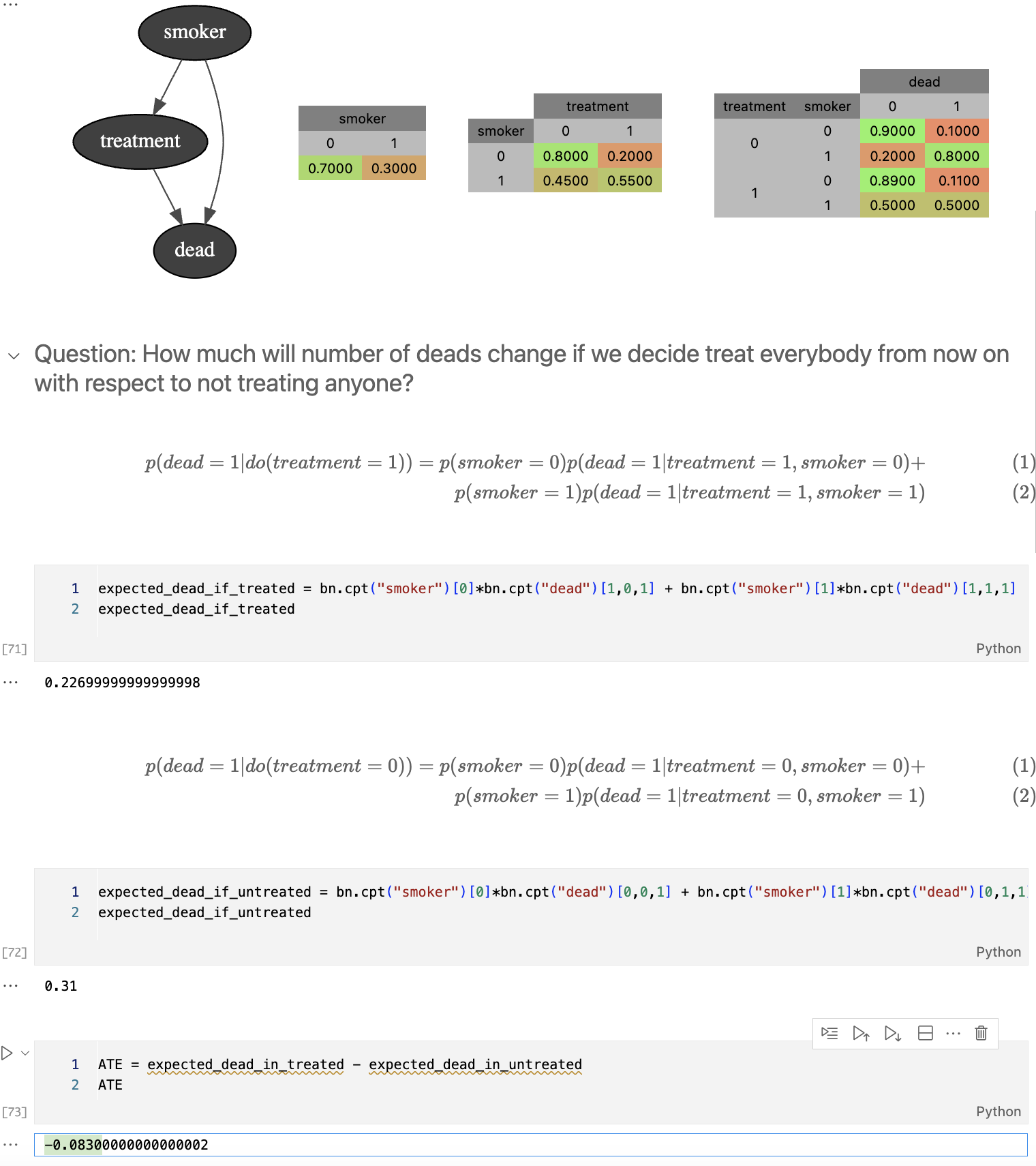
2. Propensity Score Matching
PSM은 Propensity Score를 사용하여 집단의 각 sample들을 비슷한 confounder 끼리 match해서 ATE를 계산하는 방법이다.
PSM is for cases of causal inference and confounding bias in non-experimental settings in which: (i) few units in the non-treatment comparison group are comparable to the treatment units; and (ii) selecting a subset of comparison units similar to the treatment unit is difficult because units must be compared across a high-dimensional set of pretreatment characteristics.
...
PSM employs a predicted probability of group membership—e.g., treatment versus control group—based on observed predictors, usually obtained from logistic regression to create a counterfactual group. Propensity scores may be used for matching or as covariates, alone or with other matching variables or covariates.(출처: 위키)
PSM을 사용하여 causal analysis를 하는 단계는 다음과 같다. 이에 따른 자세한 과정은 여기서 확인할 수 있다.
1. Collect data
2. Estimate propensity scores
3. Match records
4. Evaluate matching
5. Evaluate treatment effect on the outcome
(1) Pair Matching
위 데이터의 Propensity Score를 0.5 기준으로 나누고, 1000개 샘플에 대해 ATE를 계산하면 다음과 같이 $-0.074$로 정답에 꽤 가까워졌다.
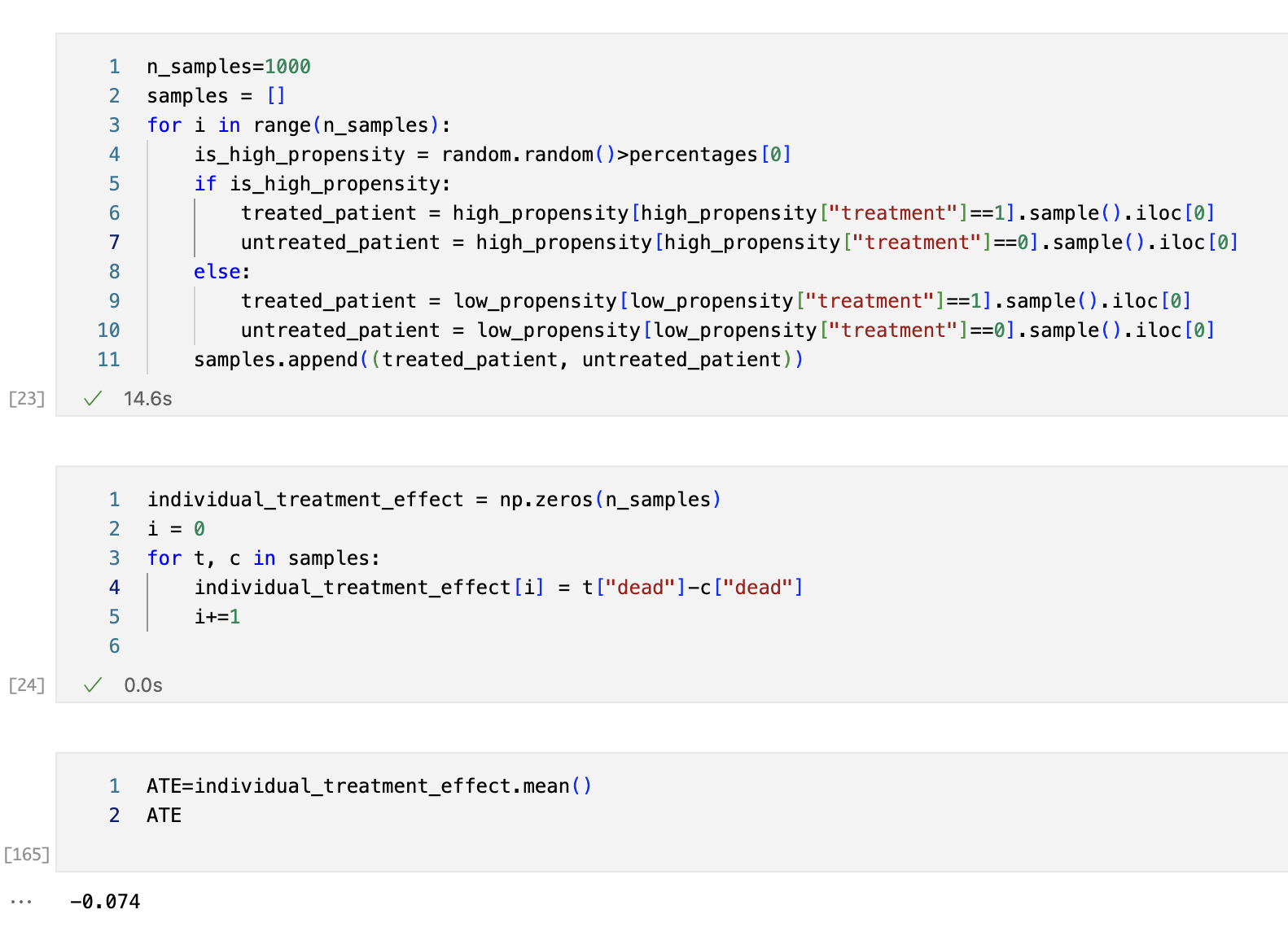
(2) Subclassification
Propensity Score의 distribution을 구간별로 나눠서 계산하는 방법이며, 다음과 같이 계산하면 정답에 매우 근사한 $-0.08304$를 얻을 수 있다.
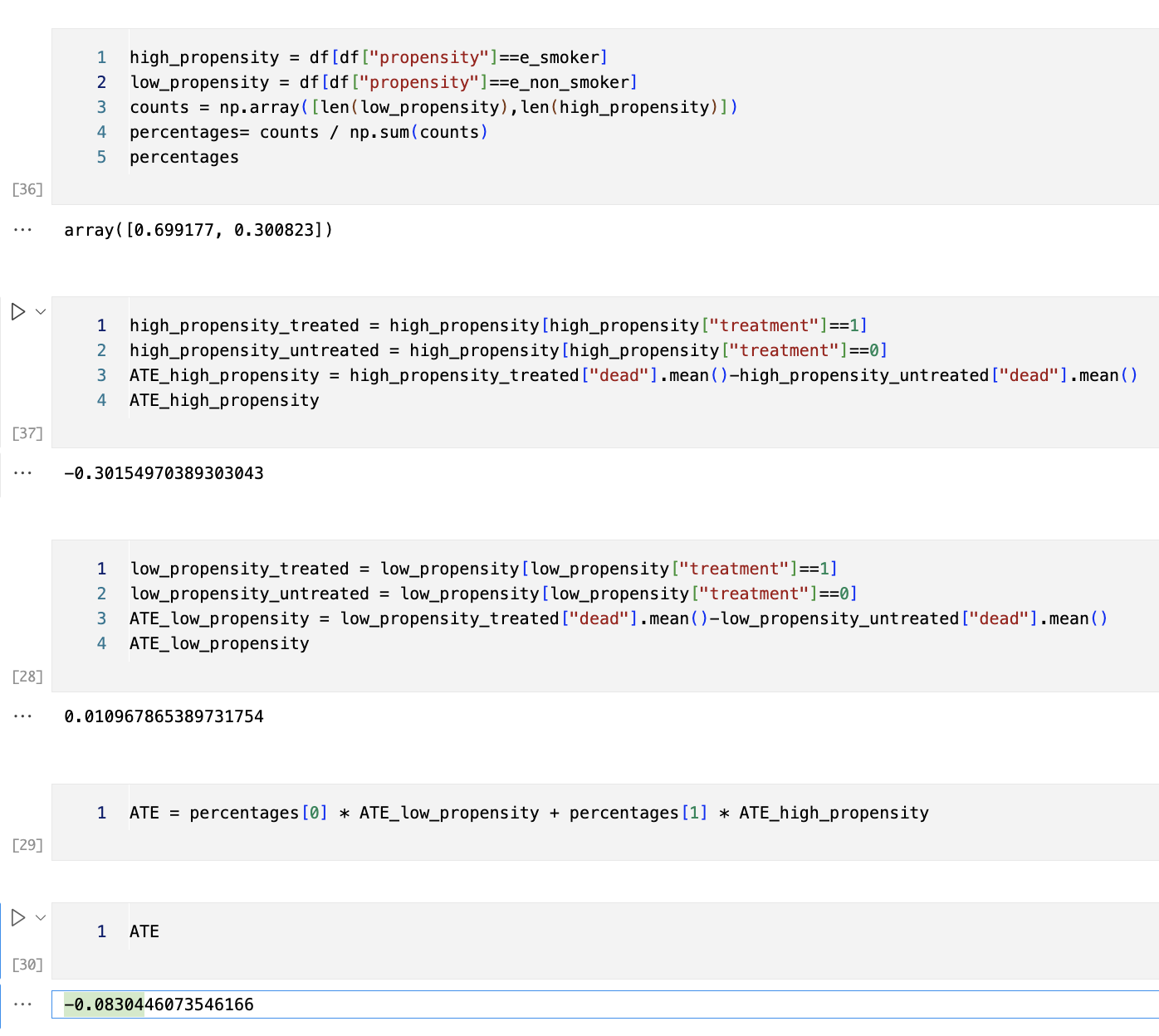
[자료출처]
- https://m.blog.naver.com/PostView.naver?isHttpsRedirect=true&blogId=chcher&logNo=220227501251
- https://builtin.com/data-science/propensity-score-matching
- https://towardsdatascience.com/a-hands-on-introduction-to-propensity-score-use-for-beginners-856302b632ac
- https://www.ncbi.nlm.nih.gov/pmc/articles/PMC4488189/
- https://github.com/cerquide/propensity_score_talk
'논문 및 개념 정리' 카테고리의 다른 글
Bio Statistics 분야에서 많이 활용되는 개념인 Propensity Score에 대해서 정리하고자 한다.
0. Propensity Score가 필요한 상황
Clinical research 분야에서 Randomized Controlled Trials(RCT)는 꽤 중요한 개념이다. 어떤 집단 A에 treatment에 대한 효과를 통계적으로 검증한다고 했을 때, 가장 이상적인 방법은 집단 A를 반으로 나눠 한쪽은 control group으로, 한쪽은 treatment를 진행한 group으로 나눠 수치를 얻어내는 것이다. 하지만 의료 분야에서는 현실적이지 않을 뿐더러 윤리적으로도 수행할 수 없는 경우가 많다. 흡연자에 대한 treatment를 검증하고자 할 때, 강제적으로 흡연을 시킬 수 없으니 말이다. 때문에 이미 실험적(experimental) 데이터가 아닌 관찰된(observational) 데이터를 주로 사용하게 된다. Propensity Score는 observational data를 가지고 causal inference를 할 때 집단간의 불균형으로 인한 bias를 보정하기 위해 사용이 된다. 그리고 보정된 값을 활용하여 Average Treatment Effect(ATE)를 계산한다.
*Experimental Data & Observational Data
Experimental data(e.g. AB test) is data collected as part of an AB test, such as a randomized experiment, controlled study or randomized control trials, among other variants. In an AB test, treatments are randomly assigned to the individuals and not selected by them. This means there is no selection bias and the treatment assignment is independent from any confounders.
Any data that is not collected as part of an AB test is observational data. In observational data, treatments are selected by the individuals and not randomly assigned to them. This means there is selection bias, and treatment is no longer independent from the confounding factors.
1. Propensity Score 개념
(1) 관련 용어 설명
Propensity Score를 설명하기 위해서 먼저 관련 용어를 정리하고자 한다.
- Outcome variable: A variable of interest for which we want to understand treatment’s effect on. This is the effect.
- Treatment variable: A variable of interest for which we want to understand its impact on the outcome variable. This is the cause.
- Confounding variable: A variable that influences both the selection of treatment and the outcome variables. In some contexts, this is also referred to as a covariate.

위와 같이 smoker, treatment, dead 항목으로 이루어진 데이터가 있다고 하자. 이때 단순히 treatment에 따른 사망 비율로 ATE를 아래와 같이 계산해보자.
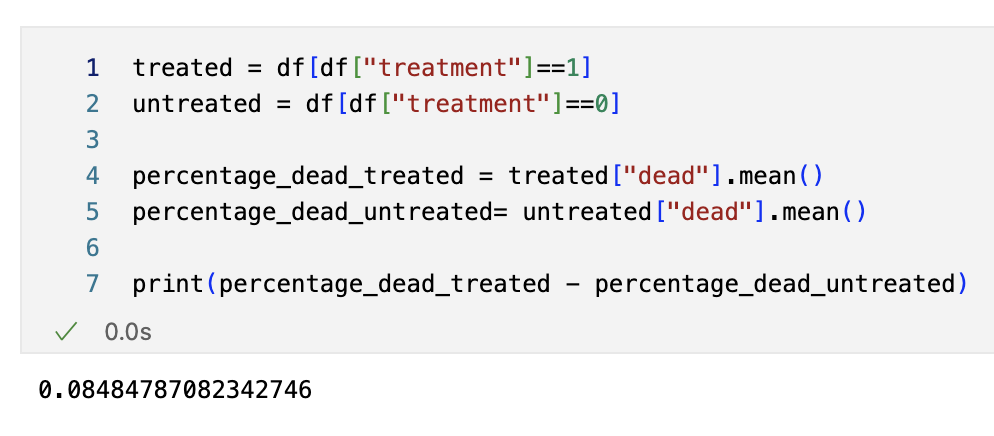
treated 집단의 사망률이 untreated 집단의 사망률보다 8.5% 높다는 결과가 나온다. 뭔가 이상하다. 이는 smoker라는 변인을 고려하지 않았기 떄문이다. Confounding variable(a.k.a covariate)은 이렇든 파악하고자 하는 cause외의 변인을 설명하기 위한 개념이다.
Basically, what this word means is that there is a variable or a group of them (the confounders) that affects in some way the probability of getting the treatment and at the same time, affects also the outcome.
하지만 confounder는 매우 많은 변인을 포함하고 있을 수 있는데, Propensity Score의 목적은 수 많은 변인들을 합쳐 하나의 score로 표현하는 것이다.
Propensity의 정의는 다음과 같다.
The "propensity" describes how likely a unit is to have been treated, given its covariate values. The stronger the confounding of treatment and covariates, and hence the stronger the bias in the analysis of the naive treatment effect, the better the covariates predict whether a unit is treated or not. By having units with similar propensity scores in both treatment and control, such confounding is reduced. (출처: 위키)
어떤 사람이 특정 confounder 변인 조합을 가지고 있을 때 treatment를 받았을 확률이다. 말이 조금 이상한데, 애초에 관찰된 데이터가 random하게 분포가 되지 않았으니, Propensity Score를 사용하여 비슷한 상황(confounder)인 사람들을 모아서 효과를 파악할 수 있다.
(2) Propensity Score 수식
ATE를 계산하기 위해서는 treated 집단과 untreated 집단의 효과 차이를 계산해야 한다.
이때
이때 Propensity Score는 다음과 같다(자세한 설명은 여기를 참고)
위의 예시 데이터로는 다음과 같이 표현할 수 있다.
Estimate of the function, often called the “propensity score,” for each and , can be obtained by fitting the parameters of a flexible function to the data at hand, in much the same way that we fitted the coefficients of a linear regression function, so as to minimize the mean square error with respect to a set of samples. The method used will depend on the nature of the random variable X, whether it is continuous, discrete or binary, for example.
위 수식을 토대로 예시 데이터의 Propensity Score를 계산하면 다음과 같다.
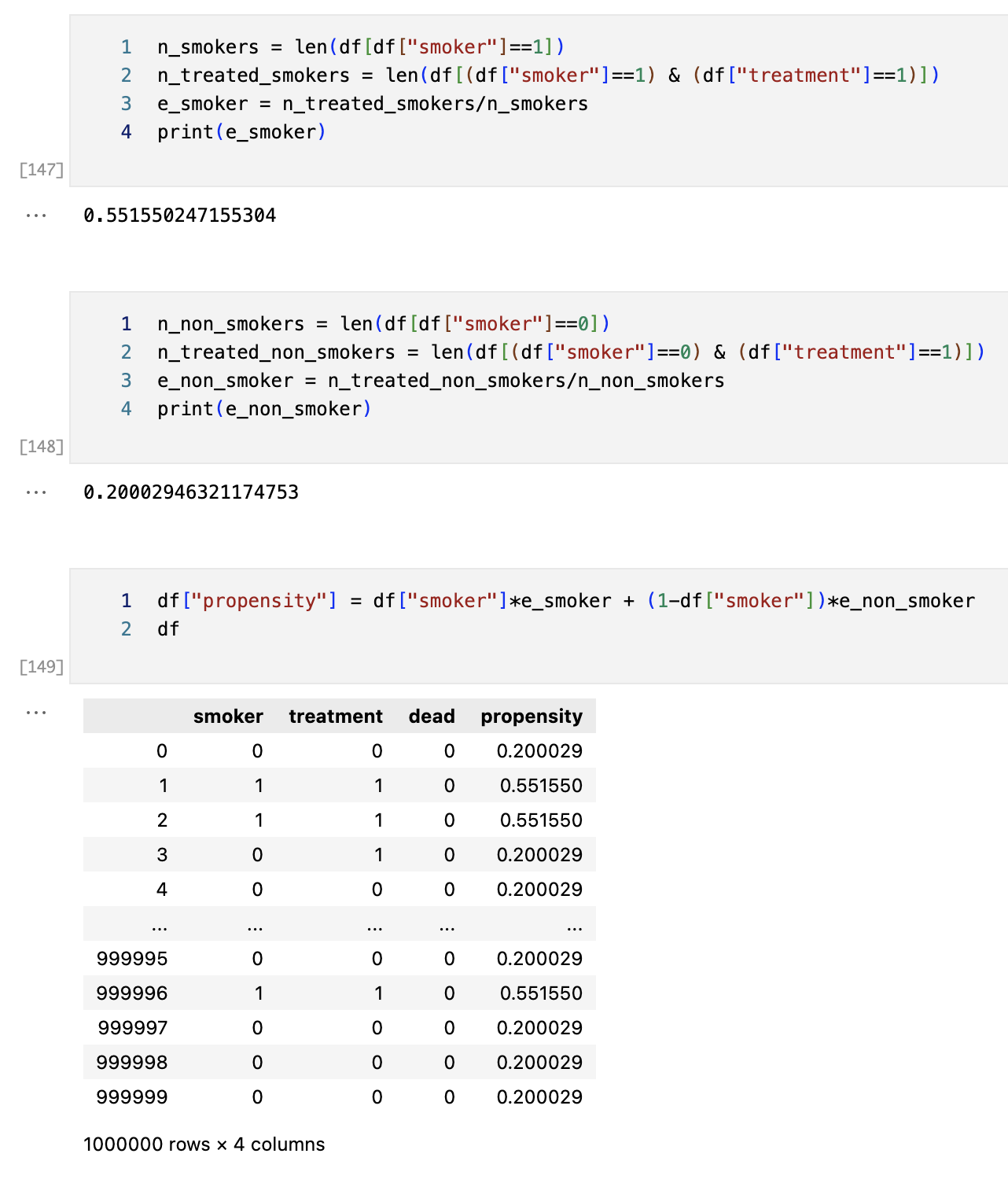
위 데이터로 ATE를 계산하면 다음과 같이
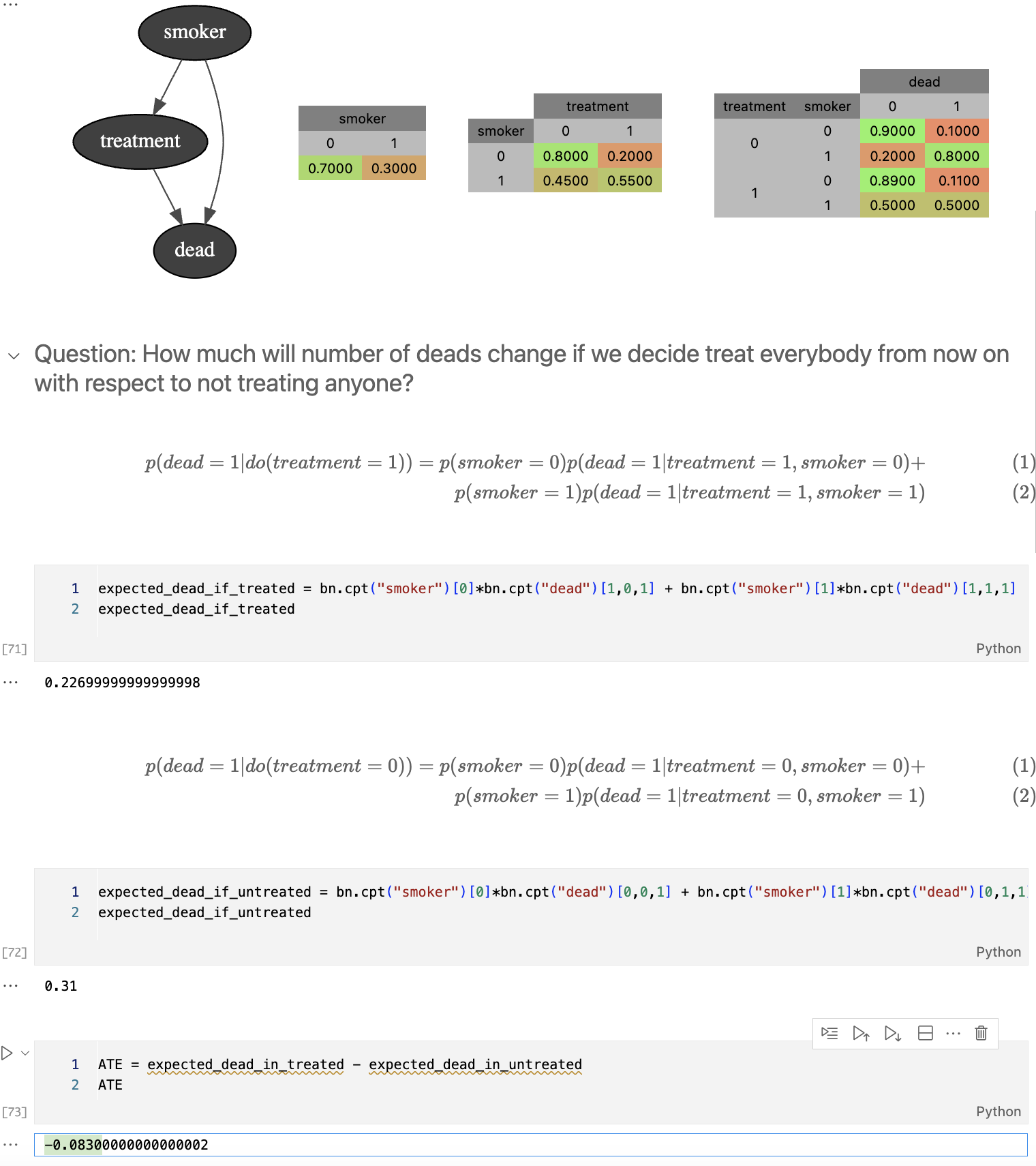
2. Propensity Score Matching
PSM은 Propensity Score를 사용하여 집단의 각 sample들을 비슷한 confounder 끼리 match해서 ATE를 계산하는 방법이다.
PSM is for cases of causal inference and confounding bias in non-experimental settings in which: (i) few units in the non-treatment comparison group are comparable to the treatment units; and (ii) selecting a subset of comparison units similar to the treatment unit is difficult because units must be compared across a high-dimensional set of pretreatment characteristics.
...
PSM employs a predicted probability of group membership—e.g., treatment versus control group—based on observed predictors, usually obtained from logistic regression to create a counterfactual group. Propensity scores may be used for matching or as covariates, alone or with other matching variables or covariates.(출처: 위키)
PSM을 사용하여 causal analysis를 하는 단계는 다음과 같다. 이에 따른 자세한 과정은 여기서 확인할 수 있다.
1. Collect data
2. Estimate propensity scores
3. Match records
4. Evaluate matching
5. Evaluate treatment effect on the outcome
(1) Pair Matching
위 데이터의 Propensity Score를 0.5 기준으로 나누고, 1000개 샘플에 대해 ATE를 계산하면 다음과 같이
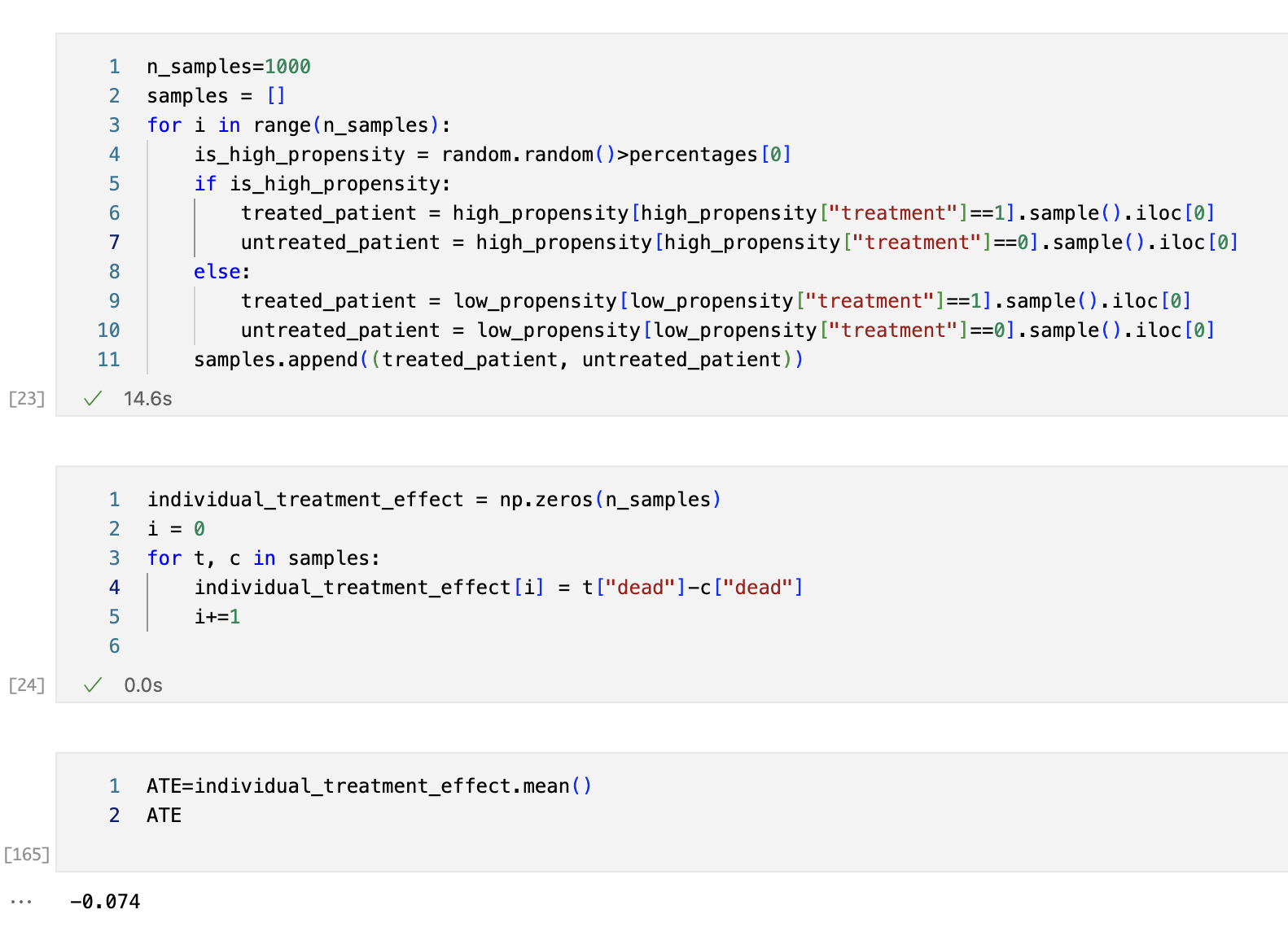
(2) Subclassification
Propensity Score의 distribution을 구간별로 나눠서 계산하는 방법이며, 다음과 같이 계산하면 정답에 매우 근사한
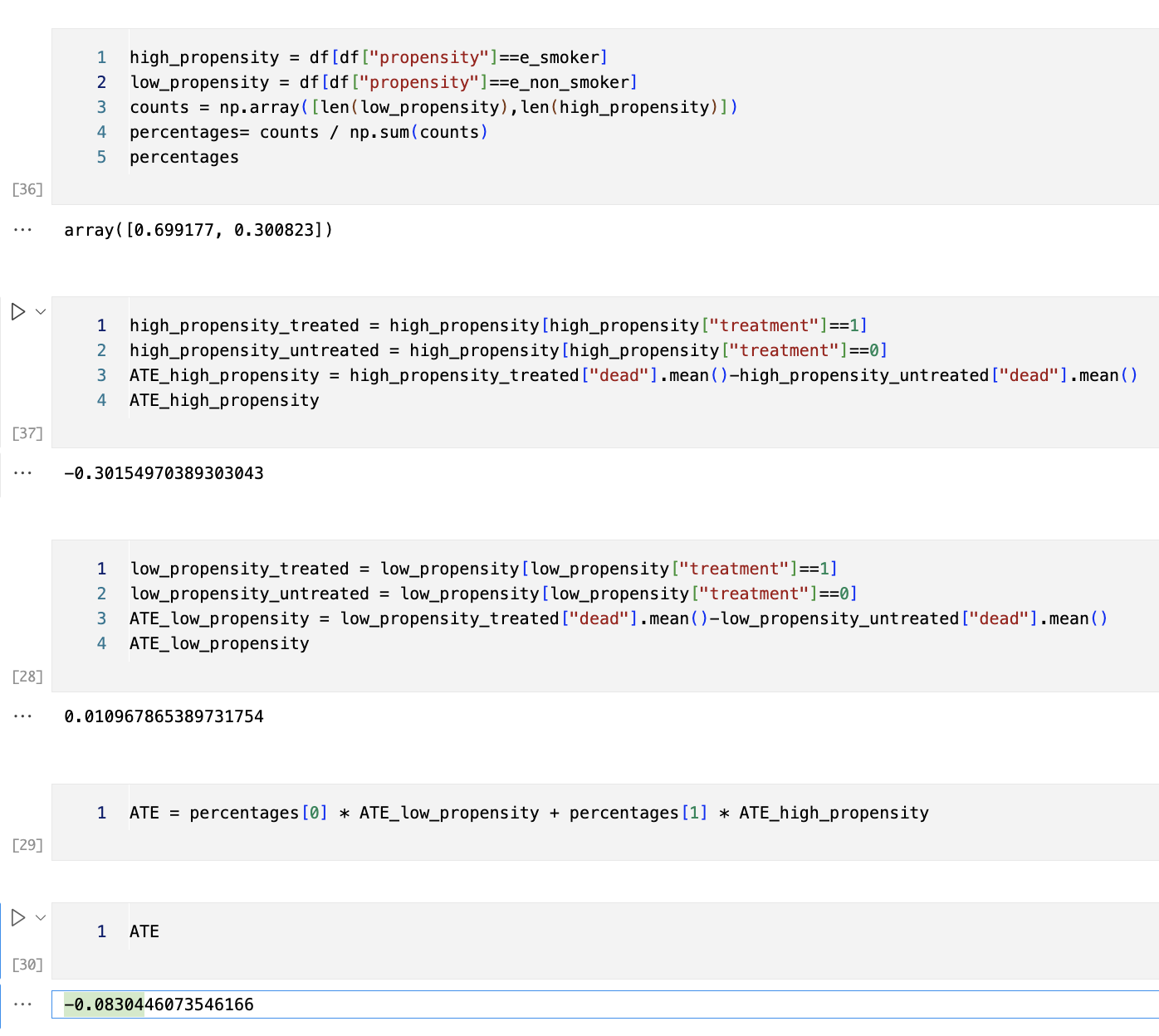
[자료출처]
- https://m.blog.naver.com/PostView.naver?isHttpsRedirect=true&blogId=chcher&logNo=220227501251
- https://builtin.com/data-science/propensity-score-matching
- https://towardsdatascience.com/a-hands-on-introduction-to-propensity-score-use-for-beginners-856302b632ac
- https://www.ncbi.nlm.nih.gov/pmc/articles/PMC4488189/
- https://github.com/cerquide/propensity_score_talk